Cancer Diagnostics (DIAG)
India Pathology Dataset (IPD)
Pathology has been at the foundation of diagnostic medicine for over a century. Significant inter- and intra-observer variability among pathologists exist. There is also a dearth of trained pathologists in India. In the era of precision medicine, accurate and timely diagnosis by pathologists is paramount for healthcare. Compelling reasons to bring in the computer-aided interpretation of histopathological images to assist pathologists. The main objectives are Creation of digital pathology datasets of Indian cohorts in two areas: oncology and nephro-pathology. Data lake for pathology, including whole slide images (WSIs) and patient's clinical characteristics, that could serve as valuable resources for research and education & develop various AI applications in pathology.
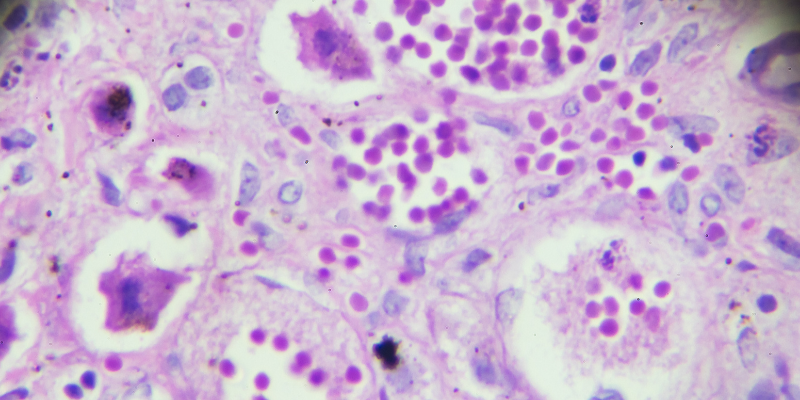
AI driven predictive models for assessing cancer probability in Oral, Breast & Lung regions
There is an increasing body of evidence that deep learning techniques can match or surpass human experts in flagging suspicious patterns found in imaging reports. This unique confluence of need and technology presents us with the opportunity to develop a way to screen early on a larger scale. To screen larger numbers of people for cancer, especially for early detection, we seek to tackle the problem by initially collecting data in photographic, DICOM formats, Annotate the data using the aid of experts: radiologists, and oncologists, Store the annotated multimodal dataset in the server and develop a model that used the data collected to predict the probability of cancer in a low resource setting.
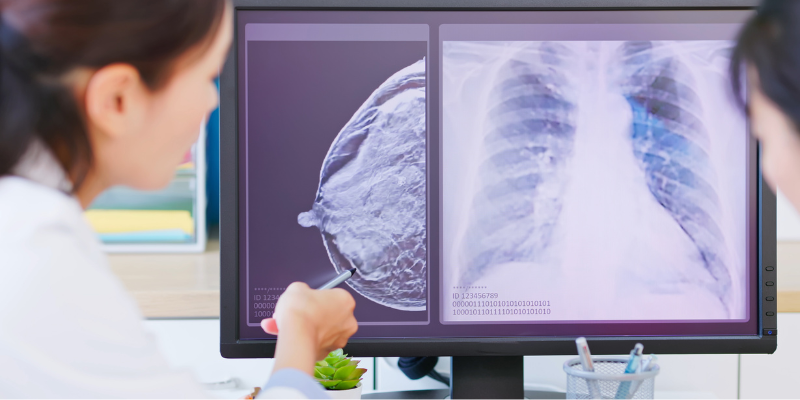
Development of Artificial Intelligence Based Solutions for Early Screening and Detection of Cervical Cancer
In this project, we propose to develop a computational framework based on deep learning to analyze the cytology images of cervical cancer. For this purpose, the whole slide images (WSIs) of pap smears will be scanned to create a digital image library. This project will be jointly done with Apollo Hospitals, Jubilee Hills, Hyderabad who will help us collect samples and provide annotations and expert guidance (phase 1). Deep learning models to detect abnormal areas and classify them into different classes: normal, inflammatory, low-grade, and high-grade squamous intraepithelial lesion, atypical glandular cells and squamous cell carcinoma based on pap smear slide images will be developed as an initial collaboration between IIIT-H and Apollo Hospitals, Jubilee Hills, Hyderabad. The total duration of project will be 24 months for creating digital image library and developing the software for screening and early detection of cervical cancer.
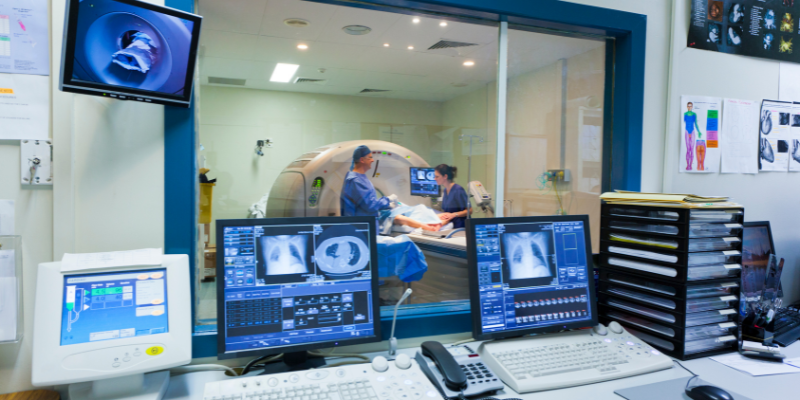
Automated scoring of HER2neu immunohistochemistry in breast cancer, with emphasis on the HER2 low subgroup
Although many studies have found, good interobserver concordance in reporting positive HER2neu cases, in light of the recent trials with emphasis on identification of HER2neu-low breast cancers, the challenge of diagnosing this category precisely for a pathologist is an uphill task. Precise assessment demands well defined guidelines and methodology to be utilized by a pathologist with specific training. Digital image analysis/ Artificial Intelligence (AI) algorithms can be utilized as a potential solution for accurate identification and quantification of the Her2neu low category. Literature search has documented improved interobserver variability by using computer aided digital microscopy. Many studies have shown the significant results with machine learning based predictions for HER2neu status and anti-HER2 treatment.
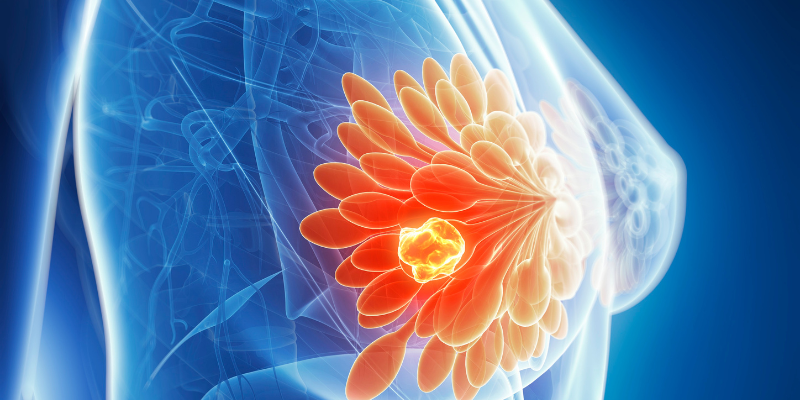